Uppsala University
Predicting moisture content in timber drying using machine learning
WISE-WASP
Open
Research question
The forest industry plays a significant role in Sweden’s economy, contributing 9–12% to national industrial employment, exports, turnover, and value added. However, it also accounts for approximately 14% of the country’s total electricity consumption. Current drying models used in sawmills are conservative, often leading to unnecessarily long drying schedules and excessive energy use. A more sustainable and economical drying process can be achieved by shortening the drying schedules while still ensuring a uniform target moisture content.
Large Swedish sawmills already employ industrial X-ray computed tomography (XCT) scanners for non-destructive strength grading, yet this technology remains underutilized in the context of drying optimization. The project aims to leverage machine learning to predict moisture content and moisture gradients based on XCT scan data. This is a crucial step toward intelligently optimizing kiln drying process.
Sustainability Aspects
The overarching goal of this project is to support the development of a timber drying process that significantly reduces energy consumption. By improving energy efficiency through data-driven insights, the project contributes to a more sustainable and environmentally responsible forest industry.
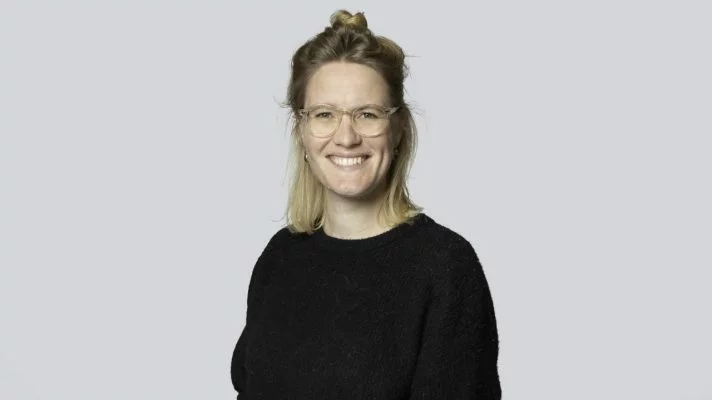
Uppsala University
Sara Florisson
Researcher
sara.florisson@angstrom.uu.se
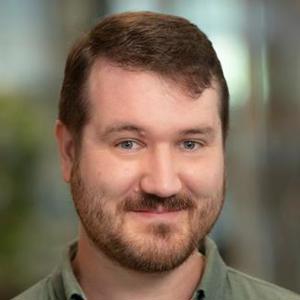
Linköping University
Sebastian Mair
Assistant Professor
sebastian.mair@liu.se
Explore projects under the WISE program
WISE drives the development of future materials science at the international forefront. The research should lead to the development of sustainable and efficient materials to solve some of today's major challenges, primary sustainability. On this page you can read more about our research projects.
Explore projects