Uppsala University
Explainable machine learning for magnetic interactions extraction
Academic project
PhD
Open
Research question
This multidisciplinary project is devoted to combining machine learning (ML) and the theory of magnetism to develop and compare methods for extracting important magnetic interactions from various types of experimental data. The project’s final goal is to identify the best method based on performance and computational complexity and use ML explainability to understand the mechanism behind the method’s performance. An important focus of the project is to address the black box problem. Specifically, most of the time, the trained ML models are treated as black boxes. However, machine learning models must be able to explain their predictions in a way people can understand. Interpretability and explainability for machine learning link input features to decision outcomes.
Sustainability aspects
The project has two main sustainability aspects. First of all, the development of new accurate ML-based methods of extracting magnetic interaction parameters can be useful for future spintronic devices, such as less energy-consuming information storage. Secondly, the developed method and a deepening understanding of the mechanism behind machine learning will be useful in general for the application of machine learning to material science.
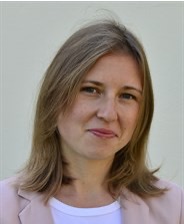
Uppsala University
Maryna Pankratova
Researcher
maryna.pankratova@physics.uu.se
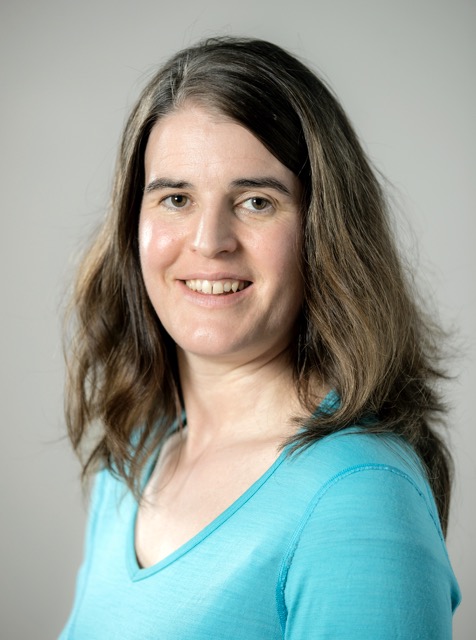
Örebro University
Stephanie Lowry
Assistant Professor
stephanie.lowry@oru.se
Explore projects under the WISE program
WISE drives the development of future materials science at the international forefront. The research should lead to the development of sustainable and efficient materials to solve some of today's major challenges, primary sustainability. On this page you can read more about our research projects.
Explore projects